Time Frequency and Wavelets in Biomedical Signal Processing 1998
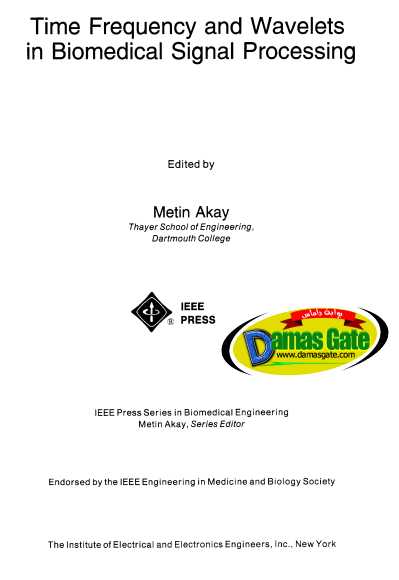
Preface
A signal can be considered to be stationary if its statistical characteristics are NOT changing with time. Stationary signals can be analyzed using classical Fourier transform methods in which the signal can be expanded on the orthogonal basis functions (sin and cosine waves). However, most biomedical signals are nonstationary and have highly complex time-fre- quency characteristics. In practice, the stationary condition for the nonstationary signals
can be satisfied by dividing the signal into blocks of short segments in which the signal segment can be assumed to be stationary. This method, called the short time Fourier trans- form (STFT) was proposed by Gabor in 1946. However, the problem with the STFT is the length of the desired segment. Choosing a short analysis window may cause poor frequency resolution. On the other hand, a long analysis window may improve the frequency resolution but compromises the assumption of stationary within the window.
To overcome these difficulties with the STFT, several time-frequency analysis methods including the Gabor representation, Wigner-Ville Distribution, Binomial transform, Choi- Williams, Reduced Interference Distribution methods etc. have been proposed. An alterna- tive way to analyze the nonstationary biomedical signals is the wavelet transform which expands the signal onto the basis functions. The basis functions can be constructed bu dilation, contractions and shifts of a unique function called the wavelet prototype. The
wavelet method act as a mathematical microscope in which we can observe different parts of the signal by just adjusting the focus. In practice, it is not necessary to the wavelet trans- form to have continuous frequency (scale) parameters, to allow fast numerical implementa-
tions, the scale can be varied only along the dyadic sequences. Therefore, the wavelet transform has a very good time resolution at the high frequencies and good frequency resolution at the low frequencies. Because of a number of theoretical a well as practical contributions made on various aspects of the WT’s, the subject is growing rapidly.
This edited book will cover the introduction to the time-frequency and wavelet trans- form methods the applications of the wavelet transforms to the biological signals including the EEG, respiratory, auditory, and evoked potential response signals etc and medical images.
Download
*
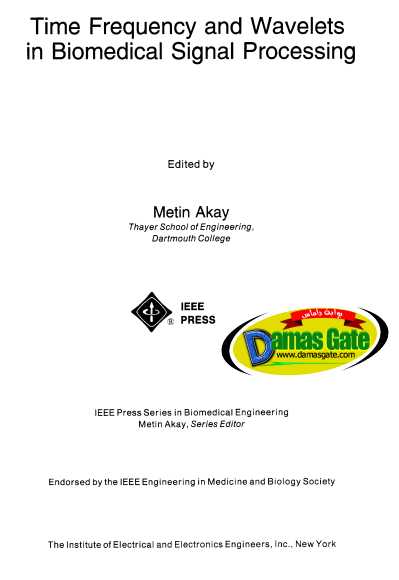
Preface
A signal can be considered to be stationary if its statistical characteristics are NOT changing with time. Stationary signals can be analyzed using classical Fourier transform methods in which the signal can be expanded on the orthogonal basis functions (sin and cosine waves). However, most biomedical signals are nonstationary and have highly complex time-fre- quency characteristics. In practice, the stationary condition for the nonstationary signals
can be satisfied by dividing the signal into blocks of short segments in which the signal segment can be assumed to be stationary. This method, called the short time Fourier trans- form (STFT) was proposed by Gabor in 1946. However, the problem with the STFT is the length of the desired segment. Choosing a short analysis window may cause poor frequency resolution. On the other hand, a long analysis window may improve the frequency resolution but compromises the assumption of stationary within the window.
To overcome these difficulties with the STFT, several time-frequency analysis methods including the Gabor representation, Wigner-Ville Distribution, Binomial transform, Choi- Williams, Reduced Interference Distribution methods etc. have been proposed. An alterna- tive way to analyze the nonstationary biomedical signals is the wavelet transform which expands the signal onto the basis functions. The basis functions can be constructed bu dilation, contractions and shifts of a unique function called the wavelet prototype. The
wavelet method act as a mathematical microscope in which we can observe different parts of the signal by just adjusting the focus. In practice, it is not necessary to the wavelet trans- form to have continuous frequency (scale) parameters, to allow fast numerical implementa-
tions, the scale can be varied only along the dyadic sequences. Therefore, the wavelet transform has a very good time resolution at the high frequencies and good frequency resolution at the low frequencies. Because of a number of theoretical a well as practical contributions made on various aspects of the WT’s, the subject is growing rapidly.
This edited book will cover the introduction to the time-frequency and wavelet trans- form methods the applications of the wavelet transforms to the biological signals including the EEG, respiratory, auditory, and evoked potential response signals etc and medical images.
Download
*