Speech, Audio, Image and Biomedical Signal Processing using Neural Networks (Studies in Computational Intelligence, Volume 83)
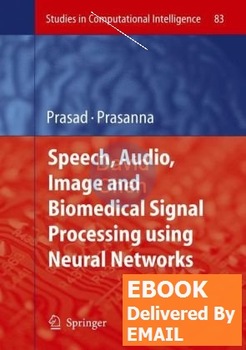
Introduction
In pattern recognition, a classifier is trained solve the multiple hypotheses testing
problem in which a particular input feature vector’s membership to one
of the classes is assessed. Given a finite number of training examples, feature
dimensionality reduction to improve generalization and optimal exploitation of
the information content in the feature vector regarding class labels is essential.
Such dimensionality reduction enables the classifier to achieve improved generalization
through (1) eliminating redundant dimensions that do not convey
reliable statistical information for classification, (2) determining a manifold
on which projections of the original high dimensional feature vector exhibit
maximal information about the class label, and (3) reducing the complexity
of the classifier to help avoid over-fitting. In other words, feature dimensionality
reduction through projections with various constraints can exploit
salient features and eliminate irrelevant feature fluctuations by representing
D. Erdogmus et al.: Information Theoretic Feature Selection and Projection, Studies in
Computational Intelligence (SCI) 83, 1–22 (2008)
Springer-Verlag Berlin Heidelberg 2008
Download
*
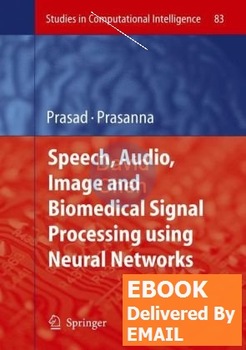
Introduction
In pattern recognition, a classifier is trained solve the multiple hypotheses testing
problem in which a particular input feature vector’s membership to one
of the classes is assessed. Given a finite number of training examples, feature
dimensionality reduction to improve generalization and optimal exploitation of
the information content in the feature vector regarding class labels is essential.
Such dimensionality reduction enables the classifier to achieve improved generalization
through (1) eliminating redundant dimensions that do not convey
reliable statistical information for classification, (2) determining a manifold
on which projections of the original high dimensional feature vector exhibit
maximal information about the class label, and (3) reducing the complexity
of the classifier to help avoid over-fitting. In other words, feature dimensionality
reduction through projections with various constraints can exploit
salient features and eliminate irrelevant feature fluctuations by representing
D. Erdogmus et al.: Information Theoretic Feature Selection and Projection, Studies in
Computational Intelligence (SCI) 83, 1–22 (2008)
Springer-Verlag Berlin Heidelberg 2008
Download
*