Computational Intelligence in Biomedical Imaging
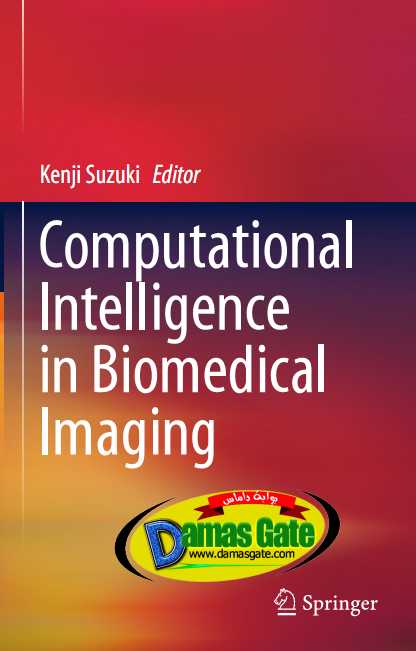
Preface
Medical decision-making is a crucial element in medicine and in patients’
healthcare; yet, it is a complex task that is often difficult even for experienced
physicians. Biomedical imaging offers useful information on patients’ medical
conditions and clues to causes of their symptoms and diseases. Thus, biomedical
imaging is indispensable for accurate decision-making in medicine. However,
physicians must interpret a large number of images. This could lead to “information
overload” for physicians, and it could further complicate medical decision-making.
Therefore, computer aids are needed and have become indispensable in physicians’
decision-making such as detection, diagnosis, and treatment of diseases.
Computational intelligence plays an essential role in computer aids for medical
decision-making, including computer-aided detection and diagnosis, computeraided
surgery and therapy, medical image analysis, automated organ/lesion segmentation,
automated image fusion, and automated image annotation and image
retrieval.
As medical imaging has been advancing with the introduction of new imaging
modalities and methodologies such as cone-beam/multi-slice computed tomography
(CT), positron-emission tomography (PET)-CT, tomosynthesis, diffusionweighted
magnetic resonance imaging (MRI), electrical-impedance tomography,
and diffuse optical tomography, new computational intelligence algorithms and
applications are needed in the field of biomedical imaging. Because of its essential
needs, computational intelligence in biomedical imaging is one of the most promising,
growing fields. A large number of researchers studied in the field and
developed a number of computational intelligence methods in biomedical imaging.
However, there has been no book that covered the state-of-the-art technologies and
recent advances in the field.
This book provides the first comprehensive overview of state-of-the-art computational
intelligence research and technologies in medical decision-making based
on biomedical images. This book covers the major technical advances and research
findings in the field of computational intelligence in biomedical imaging. Leading
researchers in the field contributed chapters to this book in which they describe their
cutting-edge studies on computational intelligence in biomedical imaging.
This book consists of three parts organized by research area in computational
intelligence in biomedical imaging: Part I deals with decision support, Part II
with computational anatomy, and Part III with image processing and analysis.
As mentioned earlier, computer aids have become indispensable in physicians’
decision-making. This books starts with the research on decision support systems.
In these systems, accurate segmentation and a precise understanding of anatomy are
crucial for improvement of the performance of decision support systems. Part II
covers this important topic, called “computational anatomy.” Image processing and
analysis are fundamental components in decision support systems as well as in
biomedical imaging. Part III deals with this indispensable topic.
Part I contains four chapters provided by leading researchers in the research area
of decision support.
In Chap. 1 in the decision support part (Part I), Drs. Cheng, Wee, Liu, Zhang, and
Shen describe a computerized brain disease classification and progression in MRI,
PET, and cerebrospinal fluid by using machine-learning classification and regression
techniques. Their study represents state-of-the-art brain research by use of
machine-learning techniques.
Chapter 2 is on content-based image retrieval (CBIR) systems based on perceptual
similarity for decision support in breast cancer diagnosis in mammography
using machine-learning algorithms by Drs. El Naqa and Yang. The authors are ones
of the pioneers who introduced and developed perceptual similarity in CBIR
systems for mammography. They also describe case-adaptive classification in
computer-aided diagnosis (CADx) for breast cancer. Their case-adaptive classification
is useful for improving the performance of a classifier in CADx.
In Chap. 3, Drs. Firjani, Khalifa, Elnakib, Gimel’farb, El-Ghar, Elmaghraby, and
El-Baz introduce computer-aided detection and diagnosis (CADe and CADx) of
prostate cancer in dynamic contrast enhanced MRI (DCE-MRI) by using image
analysis and classification techniques. The authors tackled this challenging problem
with their cutting-edge techniques.
Published: 2013-12-02 -- ISBN: 146147244X -- PDF -- 448 pages -- 16 MB
Download
*
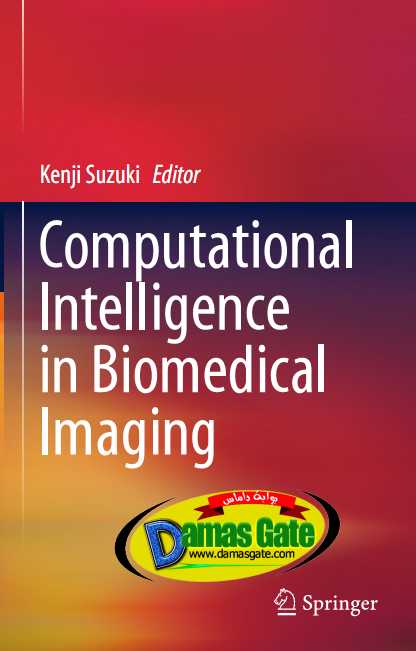
Preface
Medical decision-making is a crucial element in medicine and in patients’
healthcare; yet, it is a complex task that is often difficult even for experienced
physicians. Biomedical imaging offers useful information on patients’ medical
conditions and clues to causes of their symptoms and diseases. Thus, biomedical
imaging is indispensable for accurate decision-making in medicine. However,
physicians must interpret a large number of images. This could lead to “information
overload” for physicians, and it could further complicate medical decision-making.
Therefore, computer aids are needed and have become indispensable in physicians’
decision-making such as detection, diagnosis, and treatment of diseases.
Computational intelligence plays an essential role in computer aids for medical
decision-making, including computer-aided detection and diagnosis, computeraided
surgery and therapy, medical image analysis, automated organ/lesion segmentation,
automated image fusion, and automated image annotation and image
retrieval.
As medical imaging has been advancing with the introduction of new imaging
modalities and methodologies such as cone-beam/multi-slice computed tomography
(CT), positron-emission tomography (PET)-CT, tomosynthesis, diffusionweighted
magnetic resonance imaging (MRI), electrical-impedance tomography,
and diffuse optical tomography, new computational intelligence algorithms and
applications are needed in the field of biomedical imaging. Because of its essential
needs, computational intelligence in biomedical imaging is one of the most promising,
growing fields. A large number of researchers studied in the field and
developed a number of computational intelligence methods in biomedical imaging.
However, there has been no book that covered the state-of-the-art technologies and
recent advances in the field.
This book provides the first comprehensive overview of state-of-the-art computational
intelligence research and technologies in medical decision-making based
on biomedical images. This book covers the major technical advances and research
findings in the field of computational intelligence in biomedical imaging. Leading
researchers in the field contributed chapters to this book in which they describe their
cutting-edge studies on computational intelligence in biomedical imaging.
This book consists of three parts organized by research area in computational
intelligence in biomedical imaging: Part I deals with decision support, Part II
with computational anatomy, and Part III with image processing and analysis.
As mentioned earlier, computer aids have become indispensable in physicians’
decision-making. This books starts with the research on decision support systems.
In these systems, accurate segmentation and a precise understanding of anatomy are
crucial for improvement of the performance of decision support systems. Part II
covers this important topic, called “computational anatomy.” Image processing and
analysis are fundamental components in decision support systems as well as in
biomedical imaging. Part III deals with this indispensable topic.
Part I contains four chapters provided by leading researchers in the research area
of decision support.
In Chap. 1 in the decision support part (Part I), Drs. Cheng, Wee, Liu, Zhang, and
Shen describe a computerized brain disease classification and progression in MRI,
PET, and cerebrospinal fluid by using machine-learning classification and regression
techniques. Their study represents state-of-the-art brain research by use of
machine-learning techniques.
Chapter 2 is on content-based image retrieval (CBIR) systems based on perceptual
similarity for decision support in breast cancer diagnosis in mammography
using machine-learning algorithms by Drs. El Naqa and Yang. The authors are ones
of the pioneers who introduced and developed perceptual similarity in CBIR
systems for mammography. They also describe case-adaptive classification in
computer-aided diagnosis (CADx) for breast cancer. Their case-adaptive classification
is useful for improving the performance of a classifier in CADx.
In Chap. 3, Drs. Firjani, Khalifa, Elnakib, Gimel’farb, El-Ghar, Elmaghraby, and
El-Baz introduce computer-aided detection and diagnosis (CADe and CADx) of
prostate cancer in dynamic contrast enhanced MRI (DCE-MRI) by using image
analysis and classification techniques. The authors tackled this challenging problem
with their cutting-edge techniques.
Published: 2013-12-02 -- ISBN: 146147244X -- PDF -- 448 pages -- 16 MB
Download
*